WTI Symposium: Computation and Cognition
March 6, 2023
8:30 AM - 4:00 PM
Humanities Quadrangle, Room L01
The Wu Tsai Institute presents a Computation and Cognition symposium on Monday, March 6, at the Humanities Quadrangle on York Street. Join us in screening room L01 for a full day of talks concluding at 4:00 PM.
Breakfast will be provided, and lunch will be hosted at Mory's. Seating is limited, so please RSVP.
View the agenda and speaker details below. Contact wti@yale.edu with questions.
Agenda + Abstracts
Continental breakfast will begin at 8:30 AM, and doors will open at 8:45 AM.
Presented by Yubei Chen
Humans and other animals exhibit learning abilities and understanding of the world far beyond the capabilities of current AI and machine learning systems. Such abilities are driven largely by intrinsic objectives without external supervision. Unsupervised representation learning (aka self-supervised representation learning) aims to build models that find patterns in data automatically and reveal the patterns underlying data explicitly with a representation. Two fundamental goals in unsupervised representation learning are to model natural signal statistics and to model biological sensory systems. These are intertwined because many properties of the sensory system are adapted to the statistical structure of natural signals.
First, we can formulate unsupervised representation learning from neural and statistical principles. Sparsity provides a good account of neural response properties at the early stages of sensory processing, and low-rank spectral embedding can model the essential degrees of freedom of high-dimensional data. This approach leads to the sparse manifold transform (SMT) and offers a way to exploit the structure in a sparse code to straighten natural non-linear transformations and learn higher-order structure at later stages of processing. SMT has recently been supported by human perceptual experiments and population coding in the primary visual cortex.
Second, we can use reductionism to demonstrate that the success of state-of-the-art joint-embedding self-supervised learning methods can be unified and explained by a distributed representation of image patches. These two seemingly unrelated approaches have a surprising convergence—we can show that they share the same learning objective, and their benchmark performance can also be closed significantly. The evidence outlines an exciting direction for building a theory of unsupervised hierarchical representation and explains how the visual cortex performs hierarchical manifold disentanglement. The tremendous advancement in this field also provides new tools for modeling high-dimensional neuroscience data and other emerging signal modalities and promises unparalleled scalability for future data-driven machine learning. However, these innovations are only steps on the path to building an autonomous machine intelligence that can learn as efficiently as humans and animals. To achieve this grand goal, we must venture far beyond classical notions of representation learning.
Presented by Shreya Saxena
Our ability to record large-scale neural and behavioral data has substantially improved in the last decade. However, the inference of quantitative dynamical models for cognition and motor control remains challenging due to their unconstrained nature. Here, we incorporate constraints from anatomy and physiology to tame machine-learning models of neural activity and behavior. Saxena will show that these constraints-based modeling approaches allow us to predictively understand the relationship between neural activity and behavior. How does the motor cortex achieve generalizable and purposeful movements from the complex, nonlinear musculoskeletal system? Saxena presents a deep reinforcement learning framework for training recurrent neural network controllers that act on anatomically accurate limb models such that they achieve desired movements. We apply this framework to kinematic and neural recordings made in macaques as they perform movements at different speeds.
This framework for the control of the musculoskeletal system mimics biologically observed neural strategies and enables hypothesis generation for prediction and analysis of novel movements and neural strategies. Modeling neural activity and behavior across different subjects and in a naturalistic setting remains a significant challenge. Widefield calcium imaging enables recordings of large-scale neural activity across the mouse dorsal cortex during cognitive tasks. Here, it is critical to demix the recordings into meaningful spatial and temporal components that can be mapped onto well-defined brain regions. To this end, we developed Localized semi-Nonnegative Matrix Factorization (LocaNMF) to extract and model the activity of different brain regions in individual mice—the decomposition obtained by LocaNMF results in interpretable components which are robust across subjects and experimental conditions. Moreover, we develop novel explainable AI methods for modeling continuously varying differences in behavior, which successfully represent distinct features of multi-subject and social behavior in an unsupervised manner. These methods are also successful at uncovering the relationships between recorded neural data and the ensuing behavior.
We will take a short break between speakers.
Presented by Aran Nayebi
Deep convolutional neural networks trained on high-variation tasks ("goals") have had immense success as predictive models of the human and non-human primate visual pathways. By pairing the development of new models to solve challenging tasks with novel quantitative metrics on high-throughput neural data, we demonstrate that these initial results are examples of a more general "goal-driven" approach, yielding the most accurate models across multiple brain areas (sensory and non-sensory) and species (rodent and primate). This goal-driven approach consists of three structural and functional ingredients, which encapsulate workable hypotheses of the evolutionary constraints of the neural circuits under study. By carefully analyzing the factors contributing to model fidelity, we gain conceptual insight into the role of recurrent processing in the primate ventral visual pathway, mouse visual processing, and heterogeneity in rodent medial entorhinal cortex. The success of this more structurally and functionally tuned approach points toward the utility of goal-driven models to study natural intelligence more broadly. To this end, Nayebi sketches out the research program to design integrative agents that serve as normative accounts of how neural circuits in the brain combine to produce intelligent behavior, leading to improved, embodied AI algorithms along the way.
We will break for a private lunch at Mory’s, nearby on York Street.
Presented by Naoki Hiratani
Neural circuit architecture of diverse species often displays a remarkable degree of consistency, indicating the presence of underlying principles. Hiratani hypothesizes that the neural architectures are optimized for efficient learning and analyze the architectures of early olfactory circuits and the cerebellum. On olfactory circuits, he investigates the origin of allometric scaling laws between the size of the input and intermediate layers observed across diverse species of mammals and insects. He estimates the optimal olfactory circuits employing model selection theory and shows that the scaling laws emerge under biological constraints on the training data size and the genetic budget for hard-wiring. On the cerebellum, he investigates the functional roles of two hidden layers in the cerebellar circuit: the granule cell and Purkinje cell layers. Hiratani shows that having two hidden layers leads to a quadratic improvement of the perceptron capacity compared to a one-hidden-layer model, even under a biologically plausible learning rule. The model reveals that one-shot supervised learning between granule cells and Purkinje cells is sufficient for achieving the quadratic capacity, advancing the Marr-Albus-Ito model of the cerebellum from a deep learning perspective.
Presented by Agostina Palmigiano
Neuronal representations of sensory stimuli depend on the behavioral context and associated reward. In the mouse brain, joint representations of stimuli and behavioral signals are present even in the earliest stage of cortical sensory processing. In this work, we propose a parallel between optogenetic and behavioral modulations of activity and characterize their impact on V1 processing under a common theoretical framework.
We first infer circuitry from large-scale V1 recordings of stationary animals and demonstrate that, given strong recurrent excitation, the cell-type-specific responses imply key aspects of the known connectivity. Next, we analyze the changes in activity induced by locomotion and show that, in the absence of visual stimulation, locomotion induces a reshuffling of activity, which we describe theoretically, akin to that we had found in response to optogenetic perturbation of excitatory cells in mice and monkeys. We further find that, beyond reshuffling, additional cancellation among inhibitory interneurons needs to occur to capture the effects of locomotion. Specifically, we leverage our theoretical framework to infer the inputs that explain locomotion-induced changes in firing rates and find that, contrary to hypotheses of simple disinhibition (inhibition of inhibitory cells), locomotory drive to individual inhibitory cell types largely cancel. We show that this inhibitory cancellation is a property emerging from V1 connectivity structure.
This work is a first step towards elucidating the disparate and still poorly understood role of non-sensory signals in the sensory cortex, and uncovering the dynamical mechanisms underlying their effect. Furthermore, it establishes a foundation for future research to explore the relationship between adaptable sensory representations and cognitive flexibility.
Symposium Speakers
Yubei Chen
Intersection of computational neuroscience and deep unsupervised (self-supervised) learning, computational principles governing unsupervised representation learning in both brains and machines, natural signal statistics
Chen is a postdoctoral associate at the NYU Center for Data Science and Meta AI, working under Professor Yann LeCun. He received his MS/PhD in Electrical Engineering and Computer Sciences and MA in Mathematics at UC Berkeley under Professor Bruno Olshausen. His research interests span multiple aspects of representation learning. He explores the intersection of computational neuroscience and deep unsupervised learning, to improve our understanding of the computational principles governing unsupervised representation learning in both brains and machines and reshape our insights into natural signal statistics. He received the NSF graduate fellowship and studied electrical engineering as an undergraduate at Tsinghua University.


Shreya Saxena
Interface of statistical inference, recurrent neural networks, control theory, and neuroscience
Saxena is broadly interested in the neural control of complex, coordinated behavior. She is an Assistant Professor at the University of Florida’s Electrical and Computer Engineering Department. Before this, she was a Swiss National Science Foundation Postdoctoral Fellow at Columbia University’s Zuckerman Mind Brain Behavior Institute in the Center for Theoretical Neuroscience. She did her PhD in the Department of Electrical Engineering and Computer Science at the Massachusetts Institute of Technology, studying the closed-loop control of fast movements from a control theory perspective. Saxena received a BS in Mechanical Engineering from the Swiss Federal Institute of Technology (EPFL) and an MS in Biomedical Engineering from Johns Hopkins University. She was selected as a Rising Star in both Electrical Engineering (2019) and Biomedical Engineering (2018).


Aran Nayebi
Intersection of systems neuroscience and artificial intelligence, using tools from deep learning and large-scale data analysis to "reverse engineer" neural circuits
Nayebi is an ICoN Postdoctoral Fellow at MIT, currently working with Robert Yang and Mehrdad Jazayeri. He completed his PhD in Neuroscience at Stanford University, co-advised by Dan Yamins and Surya Ganguli. His interests lie at the intersection of systems neuroscience and artificial intelligence, where he uses tools from deep learning and large-scale data analysis to reverse-engineer neural circuits. His long-term aim is to produce normative accounts of how the brain produces intelligent behavior and to build better artificial intelligence algorithms along the way.

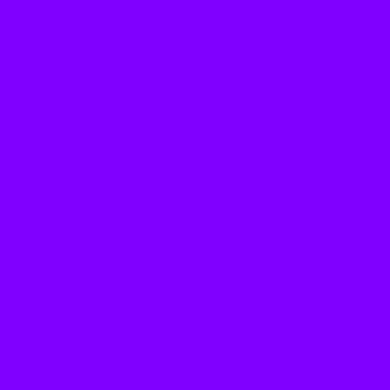
Naoki Hiratani
Bayesian synaptic plasticity, neural architecture selection, perturbation-based learning algorithms, algorithms for symbolic binding, data-efficient learning
Hiratani received his PhD from the University of Tokyo in 2016 for his work on phenomenological and normative models of synaptic plasticity and spiking neural networks at the RIKEN Brain Science Institute. He went on to work as a research fellow in the Peter Latham Lab at the Gatsby Computational Neuroscience Unit, UCL, where he investigated the development and evolution of olfactory systems and perturbation-based credit assignment mechanisms. At UCL, he also took part in the International Brain Laboratory, where he worked on the analysis of reaction time and its neural correlates. Since the end of 2020, he has been a Swartz postdoctoral fellow in the Haim Sompolinsky Lab at Harvard University, focusing on the neural substrates of cognitive functions, including binding and knowledge graph construction, as well as the development of biologically plausible credit-assignment mechanisms.
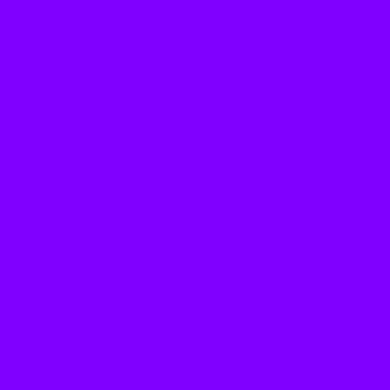
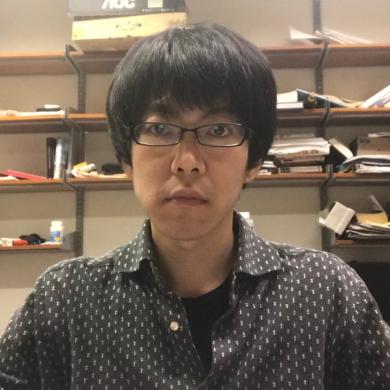
Agostina Palmigiano
Development of mathematically tractable models with biological fidelity to investigate the mechanisms underlying sensory representations and how they are shaped by and in conjunction with behavior
Palmigiano is a Simons BTI 2021 Fellow at the Center for Theoretical Neuroscience at Columbia University, working in the lab of Dr. Ken Miller. She received her BS. and MS. in physics from the University of Buenos Aires, and her PhD in theoretical neuroscience from the Max Planck Institute for Dynamics and Self-Organization, under the guidance of Dr. Fred Wolf. Her research involves the development of tractable dynamical models to investigate the mechanisms underlying flexible sensory representations and how they are shaped by and in conjunction with behavior.
