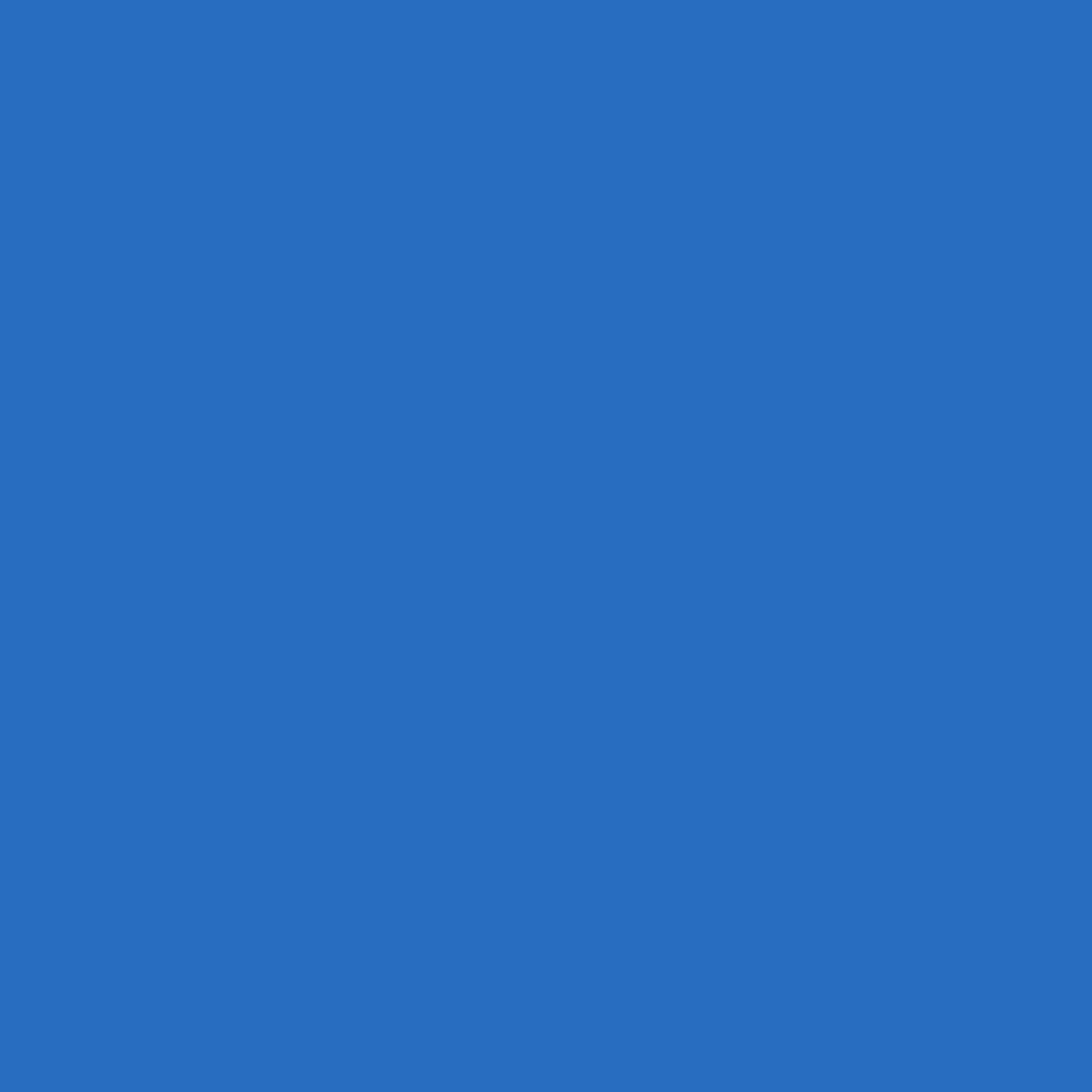
Developing efficient deep neural networks
My research is focused on bringing in priors from mathematics--geometry, topology, harmonic analysis and dynamic systems, as well as signal processing--information theory, wavelet and scattering transforms, into unsupervised learning and deep learning models in order to solve problems in biomedical and computational neuroscience. Technological revolutions have now made it possible to measure large quantities of high dimensional data on biomedical systems of all sorts, including neuronal systems. However, there is a large gap between the information inherent in this data and what can be extracted by modern machine learning methods. My research aims to bridge this gap by developing methods for unsupervised pattern detection and hypothesis generation using mathematical and data manifold priors into computationally efficient deep neural networks. Prior problems that we have tackled include manifold denoising of biomedical data to recover structure and relationships, methods for structure-preserving visualization of biomedical data in low dimensions, domain adaptation methods that can predict one datatype from another, and methods for learning high dimensional dynamics from static snapshot data. Our methods have been applied to cellular measurements from a variety of systems including cancer, immunology, neurodegeneration and developmental. More recently, we have focused on combining topological data analysis with manifold learning and deep learning in order to uncover the manifold structure and time dynamics from fMRI data of multiple participants. Future research will involve improving machine learning methods using techniques and priors that are specific to neuroscience, as well as integrating multimodal neuronal data.