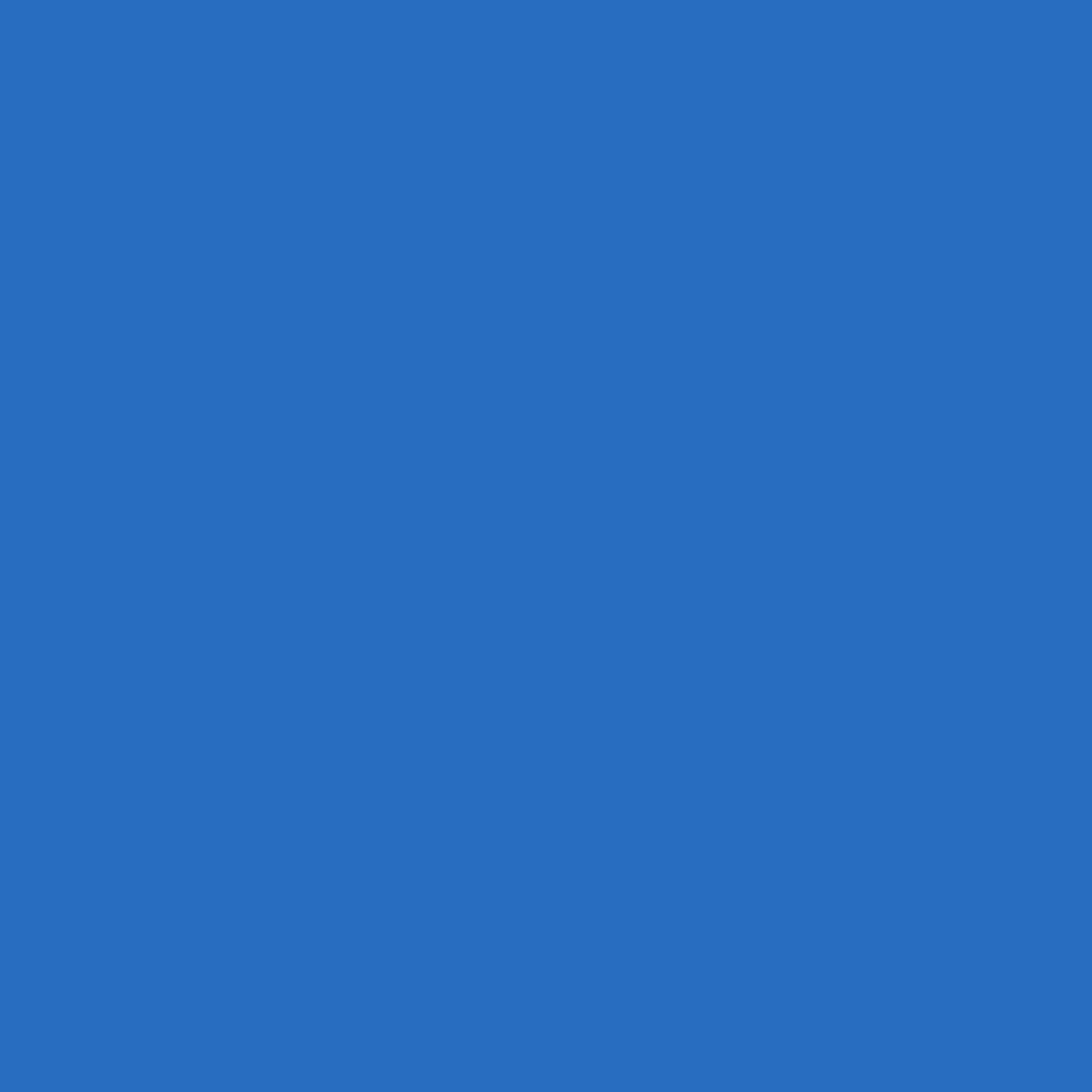
Natural language processing and deep learning
Arman Cohan's research broadly encompasses natural language processing (NLP) and artificial intelligence (AI). Language represents a crucial aspect of human cognition, enabling individuals to express thoughts, ideas, emotions, communicate with others, shape perceptions of the world, and influence thinking. The focus within NLP is on Large Language Models and Generation. The development and study of Large Language Models have direct implications for understanding human cognition, specifically in language processing. Large Language Models are a powerful tool for conducting experiments and simulations at scale, facilitating the testing of theories regarding language processing in the brain. Concurrently, insights from neuroscience, particularly concerning language acquisition and processing, contribute to developing enhanced models.
Methods
Topics
Biography
Cohan is Assistant Professor of Computer Science at Yale. His work covers a range of issues at the nexus of NLP and Machine Learning, including Language Modeling, Generation, Representation Learning, and Applications in Specialized Domains. Cohan earned his PhD from Georgetown University in 2018. Before his appointment at Yale in 2023, he was a Research Scientist at the Allen Institute for AI (AI2).